Today’s organizations have a wealth of information in various text data sources such as customer complaints, reviews and social media conversations. Most customer-centric organizations deploy text analytics tools to glean actionable insights. Still, if you ask any analyst that how often he or she was stuck massaging the data manually, the answer is typically: “Almost always.” The underlying reason for this is the complicated and contextual nature of text analytics itself.
For illustrative purposes, an airline that used a leading text analytics solution to mine customer complaints had “Upgrade to Business Class” as a key theme. This theme is not specific enough to drive any action. It is unclear whether the customers complained about upgrades that were cancelled, an inability to use miles for upgrades. or were simply saying that the upgrades were expensive. Each of these specific themes would demand a different corrective action.
Accuracy is very often a concern with text analytics tools as they cannot adequately interpret the context and nuance inherent in human language. For example, as Andrew Wilson rightly noted, most of today’s text analytics tools would classify the following statement as a negative comment about the Scion: “With the supercharger included on my Scion, it is one bad machine,” unable to recognize the colloquial use of the word “bad” to actually mean “good.”
While specificity is needed to make the themes actionable, accuracy is needed to in turn act with confidence – especially when the action is at a functional level. At the current rate of Natural Language Processing (NLP) technology advancement, it will be a long time before technology evolves to the level of human interpretation. Consequently, leading technology companies like Google and IBM have continually relied on humans to train, evaluate, edit or correct an algorithm’s work.
Organizations need to understand where NLP works best and where it doesn’t to allow for the necessary human review to complement NLP. Yes, human review can be expensive and time consuming as the scale increases; the art is in identifying the perfect combination of NLP and human review to achieve accuracy and scalability while properly managing costs.
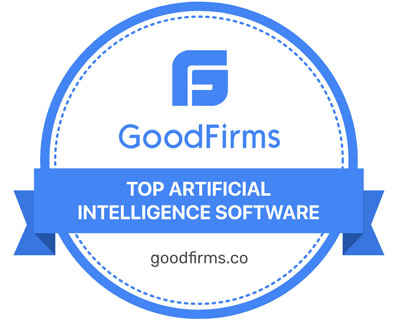