- Platform
- Solutions
Industry-specific AI solutions, while targeted, are typically modeled on generic data
- Products
- Company
About us
We are a team of data scientists, engineers and consultants committed to harnessing the vast powers of machine learning to solve distinct problems. Founded in 2012 and backed by leading investors and industry practitioners, we are experts at combining human intelligence with artificial intelligence to build custom solutions for each of our clients.
- Blog
Unstructured Customer Data Analysis using Text Mining
We build fully customized text analytics approaches to address industry specific needs, like building custom taxonomies, visualizations, models with dedicated support.
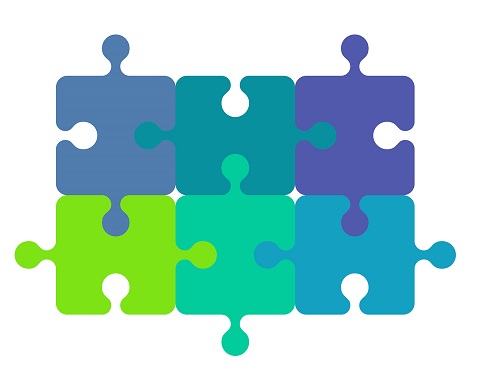
Voice of Customer Analysis – Digitalization made shopper’s lives easier in many ways – in researching products using online reviews, shopping for them on e-commerce stores and sharing their usage experiences on social channels.
User generated reviews and social comments have a significant impact on product sales. Prospective consumers use this information to make their purchase decisions. When this data is mined for specific and accurate insights, this data can offer great insight into customer sentiments and experience.
Brands can then use these insights to improve their products, marketing and customer service, which would ultimately result in higher satisfaction for consumers and better sales for the brands.
What are the sources for understanding the Voice of Customer Analytics (VoC Analysis)?
Consumers not only leave their feedback in review sites, social media, discussion forums and blogs, they also express their concerns and appreciation in private channels such as web-chat, emails, calls to customer service and customer satisfaction surveys. For most consumer focused enterprises, amount of consumer feedback can run into millions of conversations in a year.
How to get Insights from Unstructured Customer Data?
Text Mining or Text Analytics is the best approach to uncover meaningful insights from unstructured data. These analytical approaches rely on NLP (Natural Language Processing) technology. NLP is a branch of Artificial Intelligence (AI). Though this branch of AI is not as perfected as other branches of AI (e.g. Speech recognition in Apple’s Siri), with custom training, it can analyze voice of customer accurately at scale.
What are the common Challenges with Text Mining?
Off-the shelf text analytics solutions are neither accurate not provide detailed insights. This is because insights of interest vary based on use case, industry domain and data source. For most practical problems, moderate accuracy that generic text analytics solutions provide does not suffice. Also, word meaning changes based on the context. Though some of the recent advances in NLP such as word embeddings has solved this problem partially, a one-size fits all solution is still not within the reach with the current technology.
How to build cost-effective and customized Text Analytics solutions?
- Building a text analytics solution for deriving insights from voice of customer broadly involves the following steps –
- Aggregating data from disperse sources, parsing and cleaning them to the format required for the NLP models
- Evolving a taxonomy from data that fulfills the business needs of your use case
- Building a training data that accommodates the language nuances of your domain and the data you like to analyze
- Optimizing and calibrating NLP models for the precision and recall you need (i.e. accuracy and coverage)
- Setting up visualizations and analytics to answer your key business questions
These tasks require diverse skills such as data engineering, data science, human curation and analytics visualization. Most enterprises either lack the expertise or the bandwidth to do this on their own. Even for the enterprises that have the bandwidth or expertise, it would take 4 to 6 months to create such a solution.
SetuServ has built a data pipeline, annotation tools and NLP modules that can reduce the customization time to ~2 weeks and cost of customization to 20% of the cost of an in-house team. Additionally, SetuServ provides a dedicated team to provide the business support you need on an ongoing basis.